Navigating the Tech Industry: From PayPal to LinkedIn and Beyond | Mike Greenfield | Glasp Talk #9
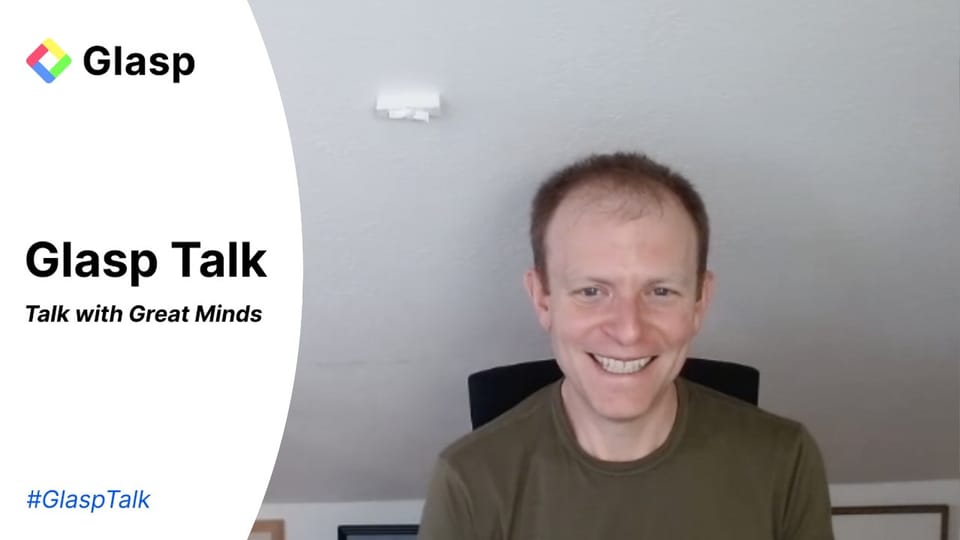
This is the ninth session of Glasp Talk!
Glasp Talk delves deep into intimate interviews with luminaries from various fields, unraveling their genuine emotions, experiences, and the stories behind them.
Today's guest is Mike Greenfield, the founder of Team Rankings, Change Research, a successful angel investor, and a former early employee at PayPal and LinkedIn.
In this interview, Mike shares his extensive experience in the data science and tech industry, discusses the evolution of data-driven decision-making, and offers valuable insights into building successful companies. He also emphasizes the importance of using data to solve complex problems and make the world a better place. Mike advises startups on leveraging data for innovation, understanding tech cycles, and overcoming challenges in various domains. Don't miss this inspiring and informative conversation!
Read the summary:
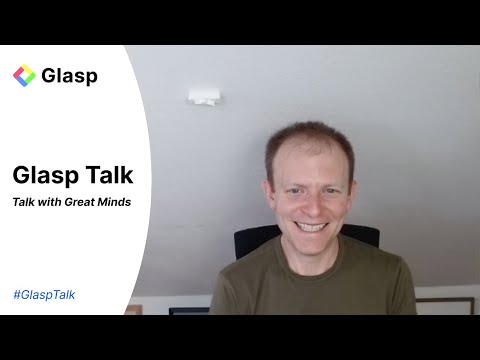
Transcripts
Glasp: Hi everyone, welcome back to Glasp Talk. Today we have an amazing guest, Mike Greenfield. Mike is a co-founder and chair of the board at Change Research. In his career, he had a bachelor's degree in Mathematical and Computational Science at Stanford back in 2000. And then he was an employee at PayPal where he built the first fraud prevention models. And then he moved on to LinkedIn, also as an early employee. If I remember correctly, Mike was among the first 10 or 15 employees at the time, right?
Mike: 15, yes.
Glasp: That's amazing! And also, he has uh invested in many successful companies, uh like Pocket, OpenDoor, and so on. So, today we'd like to ask him about his career experience and also maybe touch on AI trends, because I think he has seen many tech cycles, from the dot-com boom, mobile, AI, and so on. So, thank you for joining us today, Mike.
Mike: Thank you for having me, it's great to be talking with you both.
Glasp: First of all, uh I shared a bit about who you are and what you've done, but could you briefly introduce yourself to people who don't know you yet?
Mike: Sure, yeah. So, the way that I see my career in terms of what I've specifically worked on has been... I was a data scientist early on, working on data problems within startups, at PayPal and then at LinkedIn. I started a company in college as a side project, which was TeamRankings.com. It started as a side project for a while, but it's still around almost 25 years since I launched the website now. It’s a profitable business that has grown. But after I left LinkedIn in 2007, I really wanted to be an entrepreneur and make that who I was. So, I've been an entrepreneur, and that's been my primary focus since 2007. TeamRankings was the first company I started, and I've started a total of four different companies in fairly different domains, from sports analytics to a website for moms to, most recently, a public opinion polling company that does a large amount of work in politics. The unifying theme of all those things is the use of data to guide decision-making. I fundamentally believe that data can lead to better decisions and can make the world a better place. There are many ways that data can be used better than it is being used today, and as we collect more of it, it can be a real force for good. That's something I've devoted my career to how we create products with data and use it in better ways to both build businesses and improve the world.
Glasp: Amazing, yes, and data modeling nowadays totally makes sense. I’m curious: when did you realize you were interested in data and data science? Maybe because you went to Stanford, and at that time, you were already a "data geek" and entrepreneur in your profile, right? Were you already into data at that time, or did your interest grow over time?
Mike: Yeah, I mean, I was sort of a weird kid. This goes pretty far back. When I was 10 or so, I was reading a book called Bill James' Baseball Abstract, which looked at how data is used in baseball. This was in the mid to late 1980s, and it wasn’t a super sophisticated use of data, but I was fascinated by it. I got more into it, and sports became a significant lens of my exploration of data—baseball when I was a little kid, then later basketball. I was really into ranking teams and statistics there, and that interest just grew over time. I realized that data is a useful way to solve lots of different kinds of problems. I dabbled a bit in politics when I was in college as a political science minor, and over the past 25-plus years, I’ve worked across many fields.
Glasp: Oh, I see. So that led you to build TeamRankings at the time when you were at Stanford?
Mike: Yeah, yeah. So, what I saw, and this was in the late 90s, was that I was a big fan of college basketball at the time. Stanford had an amazing team, especially in the late 90s and early 2000s. I was pretty into that, and I noticed that the way teams were ranked relative to one another seemed arbitrary and illogical. It's a hard problem. Some sports leagues have every team play against each other, but in college basketball, there are 300+ teams playing 30 games, and some are playing almost all their games against weak opponents, and others against strong opponents. So it’s hard to tell which team is better. One team might have a losing record but plays strong opponents, while another has a winning record against weaker ones. So I started looking at how to rank teams and predict games, and that was the foundation of TeamRankings.
Glasp: That’s really interesting! Did you come up with the model by yourself, or did you read some academic papers? How did you weigh the teams?
Mike: I mostly learned by doing. There are people who are incredibly good at reading academic papers and applying them, but that’s not my strength. I think I’m good at understanding how people solve a wide range of problems and then applying those to other problems. I don’t even remember exactly how I came up with the original system for TeamRankings, but I had an understanding of linear algebra and thought maybe this linear algebra approach could apply to ranking sports teams. I brought in some additions from other sources, but it was very much a new system.
Glasp: I see. So, you started working at PayPal after that, right? How did that happen, you mentioned you built the first fraud prevention model there.
Mike: Yeah, so after I graduated from Stanford, I joined a small startup that wasn’t successful, and I was there for about eight months. While I was there, I found out that my cousin’s wife was working at PayPal. I’d heard of PayPal, and I actually signed up for it in college for the referral bonus. I was looking for jobs, and my cousin mentioned PayPal. I interviewed there, was impressed with the team, and decided to join. When I started, it took me about six months to be effective. It wasn’t a super structured environment—basically, they gave you interesting problems and told you to figure out what to do. I was 22, turning 23, and didn’t have the skills yet to navigate that well, but eventually, I built up to working on the fraud models.
Glasp: And how many people were at PayPal at the time?
Mike: I think around 100 to 150 people when I joined.
Glasp: Wow! What was it like working there as it grew?
Mike: It was a pretty intense environment. It was very no-nonsense, not a lot of politics. It was hard-driving and hardworking. Not much tolerance for 20-person meetings where nothing gets done—meetings were about getting to decisions as quickly as possible.
Glasp: That makes sense! It sounds like a fast-paced and focused culture. So, when you joined PayPal, you mentioned that you built the first fraud prevention model. Can you talk a bit more about how you developed that model and the approach you took?
Mike: Yeah, sure. So, when I started at PayPal, the fraud detection systems were fairly simple. There were rules like "If someone does this and this, we’ll flag them for potential fraud." That worked well at first, but over time, fraudsters became more sophisticated, and the rules started to lose effectiveness. I began experimenting with decision trees, which allowed us to look at individual transactions and assess the likelihood of fraud. We had a solid data set of millions of transactions and thousands of confirmed fraud cases, so I built a model based on that.
The breakthrough came when I started working with an intern who was focusing on a very specific type of fraud—merchant fraud. He was manually analyzing merchants who had just received their $2,000 in payments to see if they were likely to be fraudulent. I saw an opportunity to apply my decision tree software to his problem, and we were able to create a much more predictive model. We then scaled that model to different types of fraud across PayPal, and that became a significant part of what I worked on during my time there.
Glasp: That’s fascinating. So you spent about four years at PayPal, right? When you joined, there were around 100 people, but PayPal grew pretty quickly, especially after going public and being acquired by eBay. What was that experience like?
Mike: Yeah, PayPal grew fast, but not quite as explosively as some companies you see today. When I joined, we had about 100 to 150 employees. About 14 months after I joined, we went public, and eight months later, we were acquired by eBay. By the time we were acquired, the company had grown to maybe 600 to 800 people. Being part of eBay definitely changed the dynamic, as we were no longer a standalone company but part of a much larger organization.
Glasp: Interesting! And you mentioned earlier that PayPal and X.com were merged. Was that during your time?
Mike: That actually happened before I joined. PayPal and X.com were in the same building in 1999, and then they merged in early 2000, just before I came on board. By the time I interviewed, the company was transitioning its branding from X.com to PayPal, and I signed my offer letter when it was still technically called X.com. But I never worked with Elon Musk—he had already left as CEO before I joined.
Glasp: Oh, that’s interesting! Did you work with people like Max Levchin or Peter Thiel?
Mike: Yeah, I worked directly with Max Levchin when I first started. He was my manager for a while, and then I worked with him as part of his broader team throughout most of my time there. I didn’t have much interaction with Peter Thiel, but Max was a key figure in the company’s growth.
Glasp: That’s great! As founders ourselves, we’re curious about the culture at PayPal and what differentiates it from other startups. Was there something specific that made PayPal stand out?
Mike: PayPal’s culture was intense. It was hard-driving, and there was a lot of focus on execution. People were smart, but there wasn’t a lot of tolerance for inefficiency. Meetings were quick and to the point. If there was an issue, people would tell you directly—you wouldn’t have to deal with much politics or back-channel discussions. The focus was always on solving problems as quickly and effectively as possible. In some ways, PayPal was a tough business to build. There were many other companies at the time with better ideas or concepts, but PayPal’s strength was in execution and dealing with the challenges that came up.
Glasp: That makes sense, especially in a fast-moving environment like PayPal. So, after your time at PayPal, you moved on to LinkedIn, right? How did that transition happen?
Mike: Yeah, so while I was at PayPal, we had regular pickup soccer games, and one of the people I played soccer with was Lee Hower, who was one of the founding members of LinkedIn. Through him, I learned about LinkedIn, which had just launched a few months prior. I was interested in what they were doing and started moonlighting at LinkedIn while still working at PayPal. At the time, LinkedIn had about 250,000 users and 15 people on the team. I eventually decided to leave PayPal and join LinkedIn full-time in early 2004 because LinkedIn had all these fascinating data problems, and I wanted to be a part of that.
Glasp: That’s a pretty exciting time to join LinkedIn! What kind of data problems were you working on at the time?
Mike: The biggest data set at LinkedIn was the social network itself—connections between people. It was fascinating because it was the first time I’d worked with social network data. We were trying to figure out how people were connected to one another and how we could use those connections to provide more value. I started analyzing how people signed up for LinkedIn, how invitations were being sent out, and how we could optimize that process. We wanted to see where people were dropping off in the sign-up process and how we could improve those conversion rates. It was all about optimizing the product and getting more people to engage with the platform.
Glasp: That’s really interesting! You mentioned that LinkedIn was the first company to really leverage viral email invitations for growth. What was LinkedIn’s approach to growth at the time?
Mike: Yeah, LinkedIn was one of the first to grow through viral email invitations. We had several methods for doing this. One was allowing people to connect their Outlook address book to LinkedIn, and they could send invites to people they had in their contacts. Another method was uploading an address book from elsewhere and inviting people that way. The most common method, though, was asking people to manually invite people to LinkedIn after signing up. They’d enter the name and email of someone they wanted to connect with, and that invitation would go out. A key strategy for us was making the invite more about joining the sender’s network than joining LinkedIn. It was, “Please join my network,” not “Please join LinkedIn,” because, at the time, most people didn’t know what LinkedIn was, but they knew the person sending the invite.
Glasp: That’s a clever approach! It sounds like you were really focused on understanding how people interacted with the platform and optimizing for that. So, after three years at LinkedIn, you moved on to become an entrepreneur. What made you decide to leave LinkedIn and start your own company?
Mike: Yeah, I found the data and product aspects at LinkedIn fascinating, but I had been working on TeamRankings on the side for both my time at PayPal and LinkedIn. I really enjoyed the speed at which I could execute my own projects. LinkedIn was a bit slower moving, and I wanted to be an entrepreneur, to drive something forward myself. That was the impetus for leaving LinkedIn. I wasn’t sure what company I wanted to build when I left, but I knew I wanted to be an entrepreneur. It took about six months before I figured out what I wanted to work on.
Glasp: That’s great! What advice would you give to aspiring entrepreneurs or product managers who are looking to start their own projects or companies?
Mike: One piece of advice is to focus on discipline and steadily improving over time. Creative brilliance is important, but practice, discipline, and time spent honing your skills are often underrated. Whether it’s writing, product design, sales, or coding, the more you practice and improve over time, the more successful you’ll be. Also, don’t be afraid to start small, prototype, and iterate based on real feedback. Sometimes, what you think will work in your head won’t actually play out the way you imagined once it’s in the real world, so having that iterative mindset is crucial.
Glasp: That’s great advice, Mike. Thank you so much for joining us today and sharing your insights. It’s been really inspiring to hear about your journey from PayPal to LinkedIn and beyond.
Mike: Thank you so much, Kay and Kazuki. You guys are building something really exciting with Glasp, and I’m happy to have played a small role in it. Thanks for the thoughtful questions—it’s been a lot of fun!
Glasp: Thank you! We really appreciate it.