How will the emergence of generative AI change future products, and how should product managers respond?
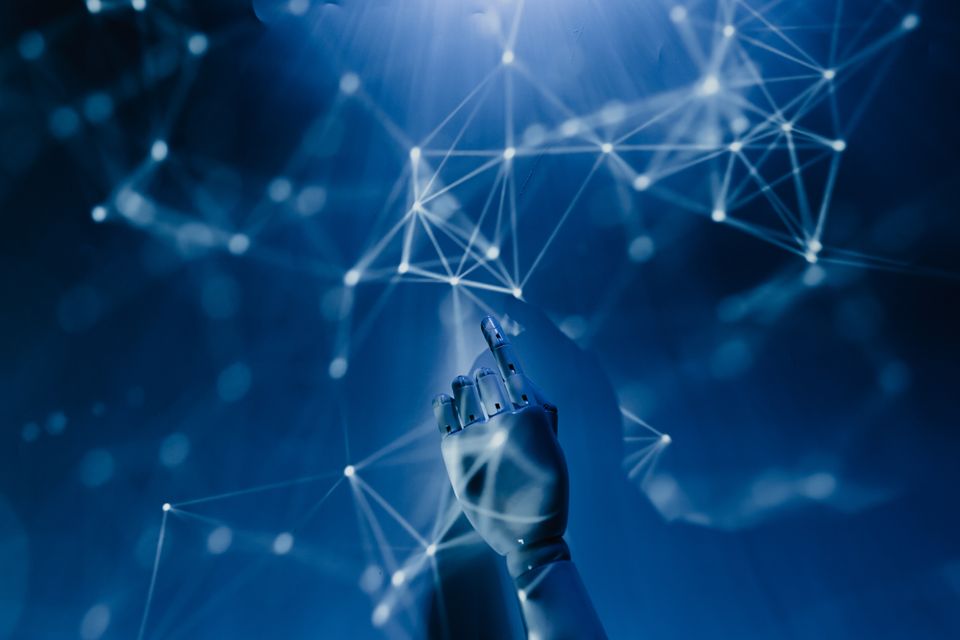
Generative AI, or "AI" is attracting a high level of attention, including "ChatGPT," which is said to have reached 100 million active users at the fastest pace in history, and "Stable Diffusion," which can automatically generate images of your choice from text. What products are starting to emerge today, where are the strengths and concerns from a business perspective, and how should product managers face them?
Introduction
The year 2022 was the year of "generative AI": who didn't see the future in products such as ChatGPT and Stable Diffusion, which allow us to have natural conversations as if we were dealing with a live person, answer our questions, and generate images simply by typing a few words? Who wouldn't? As OpenAI and other companies' AI models (i.e., AI mechanisms created by pre-training large amounts of data to return outputs in response to inputs) become more capable, they will undoubtedly have an even greater impact on society.
The adoption of generative AI will be a major paradigm shift, second only to the move to mobile and the cloud in the 2010s. In this article, I will introduce the trends of emerging AI-based products and how product managers should respond in the age of AI.
The author is a co-founder and product manager at Glasp, a startup company in San Francisco.
Trends in Emerging AI-based Products
Layers of Generative AI
Before we get into the trends of each product, let us explain the layers of generative AI. Broadly speaking, there are three layers of generative AI, from the top: the application layer, the model layer, and the cloud platform/hardware layer (there are other ways to classify the layers). For this article, the above classification will be used.
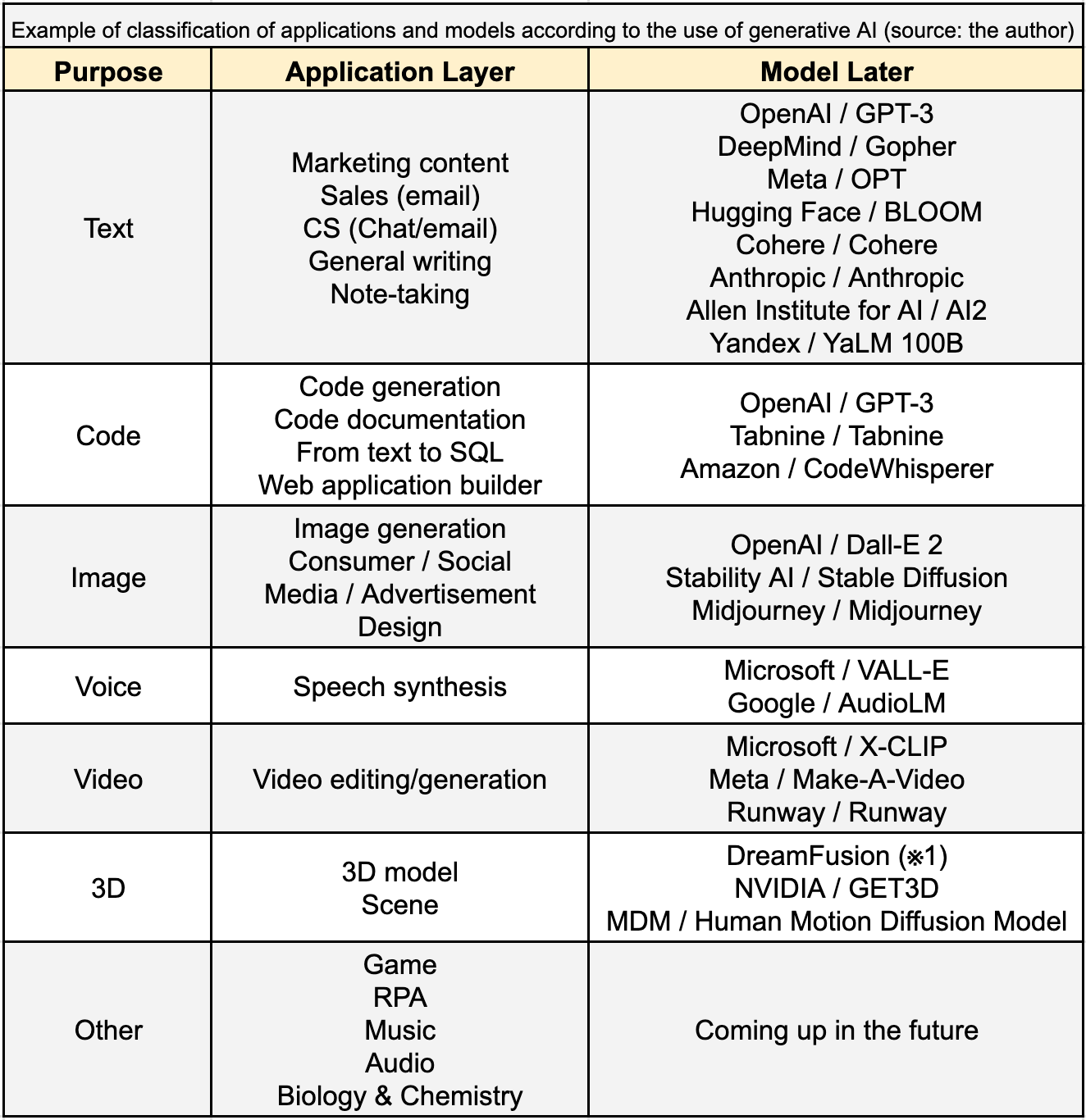
(*1) A project by researchers at Google Research and the University of California, Berkeley.
At the top is the application layer, which includes mobile and web applications that use AI models and interact with users through interfaces. You are most likely to see and hear about this layer because it can be used by ordinary users. GitHub Copilot (AI code completion tool) and Jasper (AI writing tool) are typical examples.
In the middle is the model layer. This layer contains the AI models that drive the generative AI. Typical examples are OpenAI's GPT-3, Stable Diffusion, Replicate, etc. Models like GPT-3 and Stable Diffusion are utilized to drive applications like Jasper by fine-tuning them for different purposes.
The bottom layer is the Cloud Platform/Hardware layer, where we need to process large amounts of data when creating AI models, and that is done in this layer. Details are beyond the scope of this article.
Products by Field
There have been many products using GPT-3 and Stable Diffusion. Some of the companies that were able to gain access to GPT-3 early on have already exceeded $100 million in annual recurring revenue. Based on current and historical trends, here is a list of companies and products to watch, by sector.
Among the various fields of generative AI-based products, three areas are doing relatively well. These are image generation, copywriting, and code writing. Other areas to watch are speech synthesis, video, and 3D modeling. Other areas that will be used include games, RPA, music, voice, and biochemistry.
Image Generation
The major players in this field will be Stability AI's Stable Diffusion and OpenAI's DALL-E2. OpenAI provided the model first, but since Stable Diffusion released it as open source, various products were created using Stable Diffusion. Apple has also recently updated its OS to optimize the Stable Diffusion model itself and tuned it to Apple's chips. This may provide an API for app developers to access Stable Diffusion.
Copywriting (content generation)
This area includes products for copywriting, creating content for SEO, correcting writing for writers, and suggesting what to write next. Typical examples are Jasper and Copy.ai; Jasper has annual recurring revenue of over $80 million for 2022.
Code Writing
This area includes GitHub Copilot, Tabnine, and Codeium; Codeium is free to use, while GitHub Copilot and Tabnine have already begun monetizing at $10/m and $12/m, respectively. Also, as of January 24, 1 million people have tried GitHub Copilot.
Speech Synthesis
This field includes Fliki and Murph AI. Also, podcast.ai, which created a podcast with Steve Jobs' voice, has become well-known. Compared to the above three fields, speech synthesis still seems to be in its infancy.
Video
This area includes Runway and Synthesia, which are still in the demo stage, but Synthesia is already available. What would be possible if it were possible to create a video from text?
3D Models
This area falls under GET3D, DeepMotion, etc. Many people on Twitter are already using those models to generate 3D models. The technology will be used in the film, VR, and gaming industries in the future, so it is an area that I am looking forward to seeing develop in the future.
Advantages and Disadvantages of Generative AI
Strengths of Generative AI from a Business Perspective
Before mentioning the product manager's response, I will share why generative AI is so great and the business perspective of our analysis.
Generative AI is considered revolutionary because the quality and speed of its output are overwhelming. It generates images and produces text much faster than humans, and in some areas, the quality is so high that it can be used as is.
Then there is creativity. Until now, AI has demonstrated high performance in predictive analysis, though it has not been good at being creative. For this reason, it's said that AI will replace blue-collar jobs first. However, as generative AI's creativity, it is expected to eliminate creative roles first.
Also, we shouldn't overlook that no initial data is required. In the past, AI required a large amount of data to create an AI model. Generative AI, however, provides already trained AI models, so there is no need to make AI models on your own. Thus, you can create a product related to the field without much data.
Finally, there is the cost of production. The cost of having a generative AI generate images and write code will be almost zero. The Internet revolution has reduced the marginal production and distribution costs of software products to near zero, generative AI, however, reduces the production costs to near zero. No labor costs are required. As a result, a paradigm shift is predicted.
Disadvantages/Concerns of Generative AI from a Business Perspective
On the other hand, generative AI has a number of drawbacks and concerns.
Firstly, it is difficult to maintain an advantage or strength over the competition. The advantage of generative AI is that it can make products without initial data sets, but this is the same with the ease of entry for new entrants and the difficulty for established companies to build an advantage over new entrants.
In the past, it has taken a large amount of data to create an AI product, and many companies consider holding vast amounts of data a competitive advantage. However, they can find similar data easily on the Internet. In addition, the "network effect" of data (the phenomenon in which a product or service gains additional value as more people use it) is asymptotic in nature, which means that the advantage over the competition is lost at an early stage. Also, from the user's point of view, the amount of data used to build an AI model will not be a criterion for product selection. Recently, they think of ideas for building superiority and sustainable strength, but there is no established concept yet.
Second, technical advantages are difficult to acquire: the AI model itself is open source and publicly available, and even if a product is finetuned and created, it is likely to be copied quickly and easily. The fact that many projects are open source also accelerates this point.
The third point is that speed is currently the most important factor in winning in the market. The key point is how quickly you can develop products, iterate, and create what users and customers want.
Fourth, marketing and distribution power tend to be the deciding factor in the market. And speed, financial strength, and marketing power are extremely significant factors in determining the winner.
Fifth, monetization is difficult. Even if they expand their market share, there is no efficient way to monetize their business, and we are still observing how they structure their business in the future.
How Should Product Managers Respond in the AI Era?
In this age of AI, how should product managers respond? The basic premise is that no country or product in the world will be able to escape this paradigm shift. So, instead of isolating themselves from AI, they need to quickly gather information and respond to it.
What product managers need to respond to
As mentioned above, generative AI will have a significant impact on the first three areas of text, images, and coding. The domains that will be particularly affected by these changes are design, marketing, engineering, and business models, but common points that need to be considered and addressed are as follows.
The first is the speed of product development. Generative AI is a game of speed. Again, how quickly you gather information, create the product, and take it to market is critical. As a product manager, you should focus on the necessary features and manage how quickly you can develop and launch them.
The second point is the speed of fundraising. If you are also involved in fundraising as a product manager, it is critical to move fast. The faster you can get more funding, the faster you can accelerate development, sales, and marketing, and the more likely you are to gain an advantageous position over competitors and new entrants.
The third point is the speed of sales and marketing. As generative AI moves from function to product to real business, it is safe to assume that aggressive sales and marketing, as well as AI and machine learning, will dominate 2023-2024. Aggressive sales will establish products with customers/users and give them the privilege to expand into other categories.
The fourth point is to build a competitive advantage. As discussed in the Business Perspective section, generative AI products today are at a point where it is difficult to gain an advantage over new entrants and competitors. The two ways to gain competitive advantage are network effects and embedding, as described above, but no company has succeeded in achieving strong network effects at this stage.
Finally, there are human resources. Product managers, of course, will be vital, but the key will be people who develop AI and use AI to develop products beyond what has been developed in the past. It will be necessary to actively hire people who have a deep understanding of the technology and the market and who can move quickly.
Challenges of AI
Finally, let us discuss some of the challenges of current AI. Generative AI today has some common challenges.
First, it lacks explainability. Current generative AI is based on Google's paper on large language models. It produces excellent output when run as written, but it does not explain why the output is the way it is. Researchers are currently trying to figure it out in various ways, but it will take time to figure it out. Therefore, in the unlikely event that the output is unethical, there may be problems such as uncertainty about how to deal with it and ambiguity about where responsibility lies.
Second, AI cannot determine ethics. Although ChatGPT has learned this through human interaction, it is impossible to guarantee that any issue will not happen in the future. If you use AI in your company, you need to consider the issue of AI ethics in advance and decide how to deal with it.
Finally, the accuracy of the output: models such as ChatGPT were built from the vast amount of Internet data that already exists. As such, they provide better answers than the average person for general questions. However, they are often inaccurate in specialized fields, for example. Therefore, it is necessary to consider how to ensure accuracy and in which fields to use AI.
Conclusion
Many trends and technologies have emerged in the past. And each time they have called for a paradigm shift. But the paradigm shift starting with generative AI will be far more significant than ever before. As CEOs of products, product managers need to gather information more quickly than ever before and proactively create helpful products. I hope this article has helped deepen your understanding. If you have any questions or comments, contact me on Twitter, Linkedin, or Slack.
Additional Resources
The rapid expansion of the AI product market is fostering the emergence of innovative companies and diverse career opportunities. Effectively harnessing the potential advantages and addressing the inherent challenges of AI products requires recruiting skilled AI product managers with extensive expertise in managing the complexities and factors involved. Please check it out.